Much of our thinking is flawed because it is based on faulty intuition. But by using the framework and tools of probability and statistics, we can overcome this to provide solutions to many real-world problems and paradoxes. Further and deeper exploration of paradoxes and challenges of intuition and logic can be found in my recently published book, Probability, Choice and Reason.
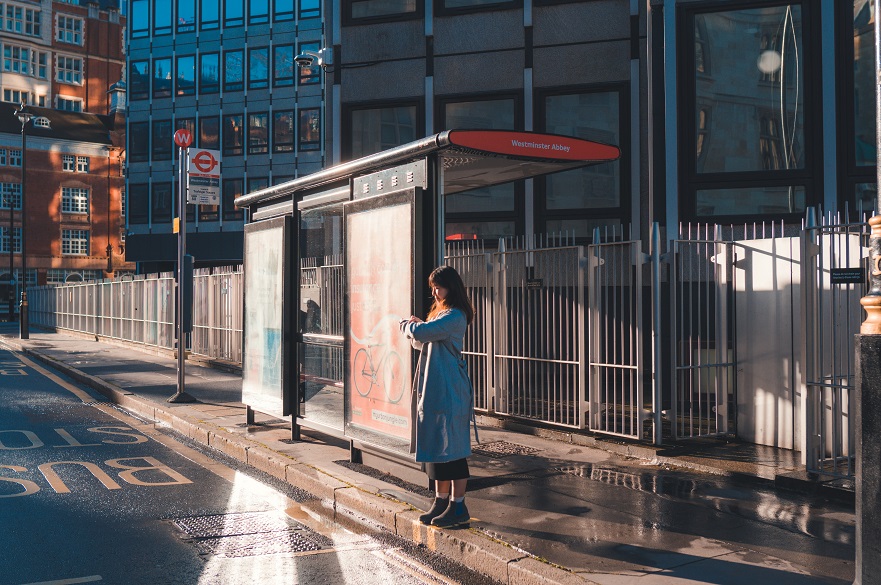
When it comes to situations like waiting for a bus, our intuition is often wrong.
Imagine, there’s a bus that arrives every 30 minutes on average and you arrive at the bus stop with no idea when the last bus left. How long can you expect to wait for the next bus? Intuitively, half of 30 minutes sounds right, but you’d be very lucky to wait only 15 minutes.
Say, for example, that half the time the buses arrive at a 20-minute interval and half the time at a 40-minute interval. The overall average is now 30 minutes. From your point of view, however, it is twice as likely that you’ll turn up during the 40 minutes interval than during the 20 minutes interval.
This is true in every case except when the buses arrive at exact 30-minute intervals. As the dispersion around the average increases, so does the amount by which the expected wait time exceeds the average wait. This is the Inspection Paradox, which states that whenever you “inspect” a process, you are likely to find that things take (or last) longer than their “uninspected” average. What seems like the persistence of bad luck is simply the laws of probability and statistics playing out their natural course.
Once made aware of the paradox, it seems to appear all over the place.
For example, let’s say you want to take a survey of the average class size at a college. Say that the college has class sizes of either 10 or 50, and there are equal numbers of each. So the overall average class size is 30. But in selecting a random student, it is five times more likely that he or she will come from a class of 50 students than of 10 students. So for every one student who replies “10” to your enquiry about their class size, there will be five who answer “50”. The average class size thrown up by your survey is nearer 50, therefore, than 30. So the act of inspecting the class sizes significantly increases the average obtained compared to the true, uninspected average. The only circumstance in which the inspected and uninspected average coincides is when every class size is equal.
We can examine the same paradox within the context of what is known as length-based sampling. For example, when digging up potatoes, why does the fork go through the very large one? Why does the network connection break down during download of the largest file? It is not because you were born unlucky but because these outcomes occur for a greater extension of space or time than the average extension of space or time.
Once you know about the Inspection Paradox, the world and our perception of our place in it are never quite the same again.
Another day you line up at the medical practice to be tested for a virus. The test is 99% accurate and you test positive. Now, what is the chance that you have the virus? The intuitive answer is 99%. But is that right? The information we are given relates to the probability of testing positive given that you have the virus. What we want to know, however, is the probability of having the virus given that you test positive. Common intuition conflates these two probabilities, but they are very different. This is an instance of the Inverse or Prosecutor’s Fallacy.
The significance of the test result depends on the probability that you have the virus before taking the test. This is known as the prior probability. Essentially, we have a competition between how rare the virus is (the base rate) and how rarely the test is wrong. Let’s say there is a 1 in 100 chance, based on local prevalence rates, that you have the virus before taking the test. Now, recall that the test is wrong one time in 100. These two probabilities are equal, so the chance that you have the virus when testing positive is 1 in 2, despite the test being 99% accurate. But what if you are showing symptoms of the virus before being tested? In this case, we should update the prior probability to something higher than the prevalence rate in the tested population. The chance you have the virus when you test positive rises accordingly. We can use Bayes’ Theorem to perform the calculations.
In summary, intuition often lets us down. Still, by applying the methods of probability and statistics, we can defy intuition. We can even resolve what might seem to many the greatest mystery of them all – why we seem so often to find ourselves stuck in the slower lane or queue. Intuitively, we were born unlucky. The logical answer to the Slower Lane Puzzle is that it’s exactly where we should expect to be!
When intuition fails, we can always use probability and statistics to look for the real answers.
Leighton Vaughan Williams, Professor of Economics and Finance at Nottingham Business School. Read more in Leighton’s new publication Probability, Choice and Reason.
There has been much discussion of late about data published on 1 November, 2021, by the Office for National Statistics (ONS). It is titled ‘Deaths involving COVID-19 by vaccination status, England: deaths occurring between 2 January and 24 September 2021’.
The raw statistics show death rates in England for people aged 10 to 59, listing vaccination status separately. https://www.ons.gov.uk/peoplepopulationandcommunity/birthsdeathsandmarriages/deaths/datasets/deathsbyvaccinationstatusengland
Counter-intuitively, these statistics show that the death rates for the vaccinated in thus age grouping were greater than for the unvaccinated. These numbers have since been heavily promoted and highlighted on social media by anti-vaccine advocates, who use them to argue that vaccination increases the risk of death.
The claim is strange, though, because we know from efficacy and effectiveness studies that COVID-19 vaccines offer strong protection against severe disease. For example, the efficiency and effectiveness of the Pfizer-BioNTech vaccine has been shown to be well over 90% in this regard in the most recent studies. https://www.yalemedicine.org/news/covid-19-vaccine-comparison
Vaccine efficacy of 90% means that you have a 90% reduced risk compared to an otherwise similar unvaccinated person, based on controlled randomised trials, while vaccine effectiveness refers to real-world outcomes. On either measure, vaccines work very well indeed.
So, what’s going on here?
Well, closer inspection of the ONS report reveals that over the period of the study, from January to September 2021, the age-adjusted risk of death involving COVID-19 was 32 times greater among unvaccinated people compared to fully vaccinated people. But hold on! How can we square this with the data from the table listing death rates of those aged 10 to 59 by vaccination status?
For the answer we turn to a classic statistical artefact known as Simpson’s Paradox, which seems to pop up and create misleading conclusions all over the place. https://leightonvw.com/2019/02/14/what-is-simpsons-paradox-and-why-it-matters/
It is a consequence of the way that data is presented.
Essentially, Simpson’s Paradox can arise when observing a feature of a broad, widely drawn group, where there is an uneven distribution of the population within this group, for example by age or vaccination status. Ignorance of the implications of Simpson’s Paradox can generate misleading conclusions, which can be, and in this case are, verydangerous.
The paradox in these particular ONS statistics arises specifically because death rates increase dramatically with age, so that at the very top end of this age band, for example, mortality rates are about 80 times as high as at the very bottom end. A similar pattern is observed between vaccination rates and age. For example, in the 10 to 59 data set more than half of those vaccinated are over the age of 40.
Those who are in the upper ranges of the wide 10 to 59 age band are, therefore, both more likely to have been vaccinated and also more likely to die if infected with COVID-19 or for any other reason, and vice versa. Age is acting, in the terminology of statistics, as a confounding variable, being positively related to both vaccination rates and death rates. Put another way, you are more likely to die in a given period if you are older and you are also more likely to be vaccinated if you are older. It is age that is driving up death rates not the vaccinations. Without the vaccinations, deaths would be hugely greater from COVID-19.
So, what if we divide the 10 to 59 group into smaller age groups?
If we break down the band into narrower age ranges, such as 10 to 19, 20 to 29, 30 to 39, 40 to 49, and 50 to 59, we find that the counter-intuitive headline finding immediately disappears. In each age band, the death rates of the vaccinated are vastly lower than those of the unvaccinated. This also applies in the higher age bands – 60 to 69, 70 to 79, and 80 plus.
Basically, unvaccinated people are much younger on average, and therefore less likely to die.
Yet there are those out there who are more than happy to use these statistics to mislead. The consequence is that many who would otherwise choose to be vaccinated might refuse to do so. In truth, the age-adjusted risk of deaths involving coronavirus (COVID-19) over the first nine months of this year was in fact 32 times greater in the unvaccinated than the fully vaccinated. This is a hugely important statistic, and we must not let statistical manipulation be used to obscure this critical information.The lives of countless people really do depend on us exposing this truth.
Leighton Vaughan Williams, Professor of Economics and Finance at Nottingham Business School. https://www.ntu.ac.uk/staff-profiles/business/leighton-vaughan-williams
Read more in Leighton’s new publication, Probability, Choice, and Reason. https://www.amazon.co.uk/Probability-Choice-Leighton-Vaughan-Williams-ebook/dp/B09DPTVFFR/ref=sr_1_2?keywords=probability+choice&qid=1638207631&qsid=262-7509985-0691032&sr=8-2&sres=3540542477%2C0367538911%2C1294977482%2C1108713505%2C1138715336%2C0521747384%2C0387715983%2C3030486001%2C1444333429%2CB07KC98Z3C%2C0071381562%2C0631183221%2C0816614407%2C1848722834%2C3319820346%2CB07SZLGZYH&srpt=ABIS_BOOK
In a fascinating article published in the New York Times, Malcolm Browne relates how Dr. Theodore Hill would ask his mathematics students to go home and either toss a coin 200 times and record the results, or else pretend that they had done so. Either way, he would ask them to produce for him the results of their (real or imaginary) coin-tossing experiment.
Dr. Hill’s purpose in this experiment was to show just how difficult it is to fake data convincingly. It just isn’t that easy to make up a random sequence. Based on this knowledge, he would astound his students by almost unerringly picking out the fakers from the tossers!
One of the ways he would do this would be to spot how many times heads or tails would be listed six or more times in a row. In real life, this occurrence is overwhelmingly probable in 200 coin throws. To most of his students this long a sequence is counter-intuitive, an example of what is often termed the Gamblers’ Fallacy, i.e. the erroneous perception that independent random sequences will balance out over time, so that for example an extended sequence of heads is more likely to be followed by a tail than a head. The fakers, susceptible to the Fallacy, are thus easily exposed. Ordinary people, even mathematics students, simply can’t help introducing patterns into what is random noise.
This is an example of a broader analysis which is usually referred to a Benford’s Law, which essentially states that if we randomly select a number from a table of real-life data, the probability that the first digit will be one particular number is significantly different to it being a different number. For example, the probability that the first digit will be a ‘1’ is about 30%, rather than the intuitive 10%, which assumes that all digits are equally likely. In particular, Benford’s Law applies to the distribution of leading and trailing digits in naturally occurring phenomena, such as the population of different countries or the heights of mountains. For example, choose a paper with a lot of numbers and circle the numbers that occur naturally, such as stock prices. So lengths of rivers lakes could be included, but not artificial numbers like telephone numbers. 30% or so of these numbers will start with a 1, and it doesn’t matter what units they are in. So the lengths of rivers could be denominated in kilometres, miles, feet, centimetres, without it making a difference to the distribution frequency of the digits.
The empirical support for this proportion can be traced to the man after whom the Law is named, physicist Dr. Frank Benford, in a paper he published in 1938, called ‘The Law of Anomalous Numbers’. In that paper he examined 20,229 sets of numbers, as diverse as baseball statistics, the areas of rivers, numbers in magazine articles and so forth, confirming the 30% rule for number 1. For information, the chance of throwing up a ‘2’ as first digit is 17.6%, and of a ‘9’ just 4.6%. The same principle applies to trailing (i.e. last) digits. It’s a great way, therefore, of checking the veracity of receipts. If, for example, there is an unusual number of trailing digit ‘7’s, there’s a decent chance that the figures are cooked.
To explain the basis of Benford’s Law, take £1 as a base. Assume this now grows at 10% per day.
£1.10, £1.21, £1.33, £1.46, £1.61, £1.77, £1.94, £2.14, £2.35, £2.59, £2.85, £3.13, £3.45, £3.80, £4.18, £4.59, £5.05, £5.56, £6.11, £6.72, £7.40, £8.14, £8.95, £9.84, £10.83, £11.92, £13.11, £14.42, £15.86, £17.45, £19.19, £21.11, £23.22, £25.50, £28.10, £30.91, £34.00, £37.40, £41.14, £45.26, £49.79, £54.74, £60.24, £72.89, £80.18, £88.20, £97.02 …
So we see that the numbers stay a long time in the teens, less in the 20s, and so on through the 90s, and this pattern continues through three digits and so forth. Benford noticed that the probability that a number starts with n = log (n+1) – log (n).
NB log10 1 = 0; log10 2 = 0.301; log10 3 = 0.4771 … log10 10 = 1.
Leading digit Probability
• 1 30.1%
• 2 17.6%
• 3 12.5%
• 4 9.7%
• 5 7.9%
• 6 6.7%
• 7 5.8%
• 8 5.1%
• 9 4.6%
Tax authorities are alert to this, or should be, which should make fraudulent activity just that little bit easier to detect, especially when the fraudster is unaware of the Benford distribution. For all right-minded citizens, we can call that Benford’s Bonus.
Links:
http://www.rexswain.com/benford.html
http://www.jstor.org/pss/984802
Further and deeper exploration of paradoxes and challenges of intuition and logic can be found in my recently published book, Probability, Choice and Reason.
Ask someone to toss a fair coin 32 times. Which of the following rows of coin toss patterns is more likely to result if they actually do toss the coins and record them accurately, and which is likely to be the fake?
HTTHTHTTHHTHTHHTTTHTHTTHTHHTTHHT
OR
HTTHTHTTTTTHTHTTHHHHTTHTHTHHTHHT
In both cases, there are 15 heads and 17 tails.
But would we expect a run (r) of five Heads or a run of five tails in the series, where r is the length of the run?
The chance of five heads = (1/2) to the power of r = (1/2) to the power of 5 = 1/32. But there are 28 opportunities for a run of five heads in 32 tosses. Same for a run of five tails.
A good rule of thumb is that when N (the number of opportunities for a run to take place) x (1/2 to the power of r) equals 1, it is likely that a run of length, r, will appear in the sequence. So, a run of length r is likely to appear when N = 2 to the power of r.
In the case of 32 coin tosses, with 28 possible runs of length five, N (28) is almost equal to 2 to the power of 5 (32). So a run of five heads (or of tails) is likely if a fair coin is tossed randomly 32 times in a row, and a run of four is almost certain.
Now look at the series of coin tosses above. The first series of 32 coin tosses has no run of heads (or tails) longer than three. The second series has a run of five tails and of four heads.
It is very likely indeed, therefore, that the second series is the genuine one, and the first one is the fake.
Appendix
Probability of 5 heads in a row = 1/32.
Probability of NOT getting 5 heads in a row from a particular run of 5 coin tosses = 31/32
Chance of NOT getting 5 heads in a row from 28 runs of five coin tosses = (31/32) to the power of 28 = 41.1%.
Therefore, the probability of getting 5 heads in a row from 28 runs of five coin tosses = 58.9%.
Similarly for tails.
The Probability of 5 heads OR 5 tails in a row = 1/32 + 1/32 = 1/16
Probability of NOT getting 5 heads OR 5 tails in a row from a particular run of 5 coin tosses = 15/16
Chance of NOT getting 5 heads OR 5 tails in a row from 28 runs of five coin tosses = (15/16) to the power of 28 =16.4%.
Therefore, the probability of getting 5 heads OR 5 tails in a row from 28 runs of five coin tosses = 83.6%
Probability of 4 heads in a row = 1/16.
Probability of NOT getting 4 heads in a row from a particular run of 4 coin tosses = 15/16
Chance of NOT getting 4 heads in a row from 29 runs of four coin tosses = (15/16) to the power of 29 = 15.4%.
Therefore, the probability of getting 5 heads in a row from 28 runs of five coin tosses = 84.6%.
Similarly for tails.
Probability of 4 heads OR 4 tails in a row = 1/16 + 1/16 = 1/8
Probability of NOT getting 4 heads OR 4 tails in a row from a particular run of 4 coin tosses = 7/8
Chance of NOT getting 4 heads OR 4 tails in a row from 29 runs of four coin tosses = (7/8) to the power of 29 = 2.1%
Therefore, the probability of getting 4 heads OR 4 tails in a row from 29 runs of four coin tosses = 97.9%
Exercise
When Nasser Hussain was England cricket captain during 200-01, he lost all 14 coin tosses in the international matches he captained. Given that he captained England in all international matches about a hundred times, what was the probability that he would face this long a losing streak during his captaincy?
Further and deeper exploration of paradoxes and challenges of intuition and logic can be found in my recently published book, Probability, Choice and Reason.
A viscountess, a radio DJ, a reality star, a vlogger, a comedian, several sportspeople and an assortment of actors and presenters. These, more or less, are the celebrities lined up to compete in the 2019 season of Strictly Come Dancing.
Outside their day jobs, few people know much about them yet. But over the 13 weeks or so of shows up until Christmas, viewers will at least learn how well the contestants can dance. But how much will their success in the competition have to do with their foxtrot and to what extent will it be, literally, the luck of the draw that sees the victors lift the trophy in December?
A seminal study published in 2010 looked at public voting at the end of episodes of the various Idol television pop singing contests and found that singers who were later on in the bill got a disproportionately higher share of the public vote than those who had preceded them.
This was explained as a “recency effect” – meaning that those performing later are more recent in the memory of people who were judging or voting. Interestingly, a different study, of wine tasting, suggested that there is also a significant “primacy effect” which favours the wines that people taste first (as well, to some extent, as last).
A little bias is in order
What would happen if the evaluation of each performance was carried out immediately after each performance instead of at the end – surely this would eliminate the benefit of going last as there would be equal recency in each case? The problem in implementing this is that the public need to see all the performers before they can choose which of them deserves their vote.
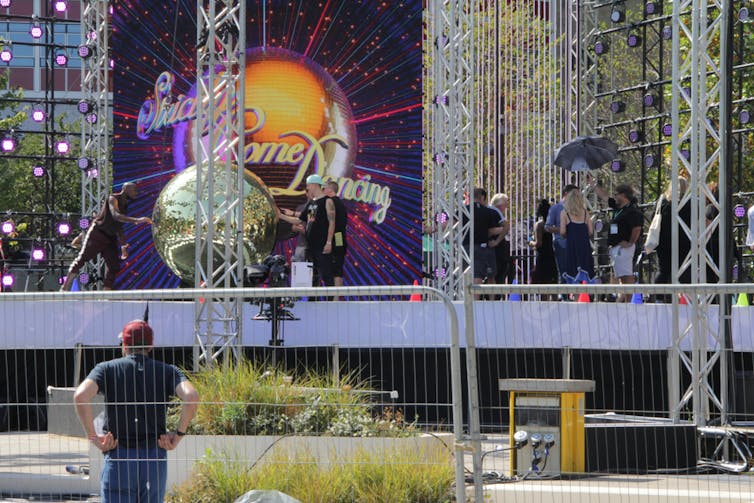
You might think the solution is to award a vote to each performer immediately after each performance – by complementing the public vote with the scores of a panel of expert judges. And, of course, Strictly Come Dancing (or Dancing with the Stars if you are in the US) does just this. So there should be no “recency effect” in the expert voting – because the next performer does not take to the stage until the previous performer has been scored.
We might expect in this case that the later performers taking to the dance floor should have no advantage over earlier performing contestants in the expert evaluations – and, in particular, there should be no “last dance” advantage.
We decided to test this out using a large data set of every performance ever danced on the UK and US versions of the show – going right back to the debut show in 2004. Our findings, published in Economics Letters, proved not only surprising, but almost a bit shocking.
Last shall be first
Contrary to expectations, we found the same sequence order bias by the expert panel judges – who voted after each act – as by the general public, voting after all performances had concluded.
We applied a range of statistical tests to allow for the difference in quality of the various performers and as a result we were able to exclude quality as a reason for getting high marks. This worked for all but the opening spot of the night, which we found was generally filled by one of the better performers.
So the findings matched the Idol study in demonstrating that the last dance slot should be most coveted, but that the first to perform also scored better than expected. This resembles a J-curve where there are sequence order effects such that the first and later performing contestants disproportionately gained higher expert panel scores.
Although we believe the production team’s choice of opening performance may play a role in this, our best explanation of the key sequence biases is as a type of “grade inflation” in the expert panel’s scoring. In particular, we interpret the “order” effect as deriving from studio audience pressure – a little like the published evidence of unconscious bias exhibited by referees in response to spectator pressure. The influence on the judges of increasing studio acclaim and euphoria as the contest progresses to a conclusion is likely to be further exacerbated by the proximity of the judges to the audience.
When the votes from the general public augment the expert panel scores – as is the case in Strictly Come Dancing – the biases observed in the expert panel scores are amplified. All of which means that, based on past series, the best place to perform is last and second is the least successful place to perform.
The implications of this are worrying if they spill over into the real world. Is there an advantage in going last (or first) into the interview room for a job – even if the applicants are evaluated between interviews? The same effects could have implications in so many situations, such as sitting down in a dentist’s chair or doctor’s surgery, appearing in front of a magistrate or having your examination script marked by someone with a huge pile of work to get through.
One study, reported in the New York Times in 2011, found that experienced parole judges granted freedom about 65% of the time to the first prisoner to appear before them on a given day, and the first after lunch – but to almost nobody by the end of a morning session.
So our research confirms what has long been suspected – that the order in which performers (and quite possibly interviewees) appear can make a big difference. So it’s now time to look more carefully at the potential dangers this can pose more generally for people’s daily lives, and what we can do to best address the problem.
Further and deeper exploration of paradoxes and challenges of intuition and logic can be found in my recently published book, Probability, Choice and Reason.
The bus arrives every twenty minutes on average, though sometimes the interval between buses is a bit longer and sometimes a bit shorter. Still, it’s 20 minutes taken as an average, or an average of three buses an hour. So you emerge onto the main road from a side lane at some random time, and come straight upon the bus stop. How long can you expect to wait on average for the next bus to arrive?
The intuitive answer is 10 minutes, since this is exactly half way along the average interval between buses, and if your usual wait is rather longer than this, then you have been unlucky.
But is this right? The Inspection Paradox suggests that in most circumstances you will actually be quite lucky only to wait ten minutes for the next bus to arrive.
Let’s examine this more closely. The bus arrives every 20 minutes on average, or three times an hour on average. But that is only an average. If they actually do arrive at exactly 20 minute intervals, then your expected wait is indeed 10 minutes (the mid-point of the interval between the bus arrivals). But if there is any variation around that average, things change, for the worse.
Say for example, that half the time the buses arrive at a ten minute interval and half the time at a 30 minute interval. The overall average is now 20 minutes, but from your point of view it is three times more likely that you’ll turn up during the 30 minute interval than during the ten minute interval. Your appearance at the stop is random, and as such is more likely to take place during a long interval between two buses arriving than during a short interval. It is like randomly throwing a dart at a timeline 30 minutes long. You could well hit the ten minute interval but it is much more likely that you will hit the 30 minute interval.
So let’s see what this means for our expected wait time. If you randomly arrive during the long (30 minute) interval, you can expect to wait 15 minutes. If you randomly arrive during the short (10 minute) interval, you can expect to wait 5 minutes. But there is three times the chance you will arrive during the long interval, and therefore three times the chance of waiting 15 minutes as five minutes. So you expected wait is 3×15 minutes plus 1x 5 minutes, divided by four. This equals 50 divided by 4 or 12.5 minutes.
In conclusion, the buses arrive on average every 20 minutes but your expected wait time is not half of that (10 minutes) but more in every case except when the buses arrive at exact 20 minute intervals. The greater the dispersion around the average, the greater the amount by which your expected wait time exceeds the average wait time. This is the ‘Inspection Paradox’, which states than whenever you ‘inspect’ a process you are likely to find that things take (or last) longer than their ‘uninspected’ average. What seems like the persistence of bad luck is actually the laws of probability and statistics playing out their natural course.
Once made aware of the paradox, it seems to appear everywhere.
For example, take the case where the average class size at an institution is 30 students. If you decide to interview random students from the institution, and ask them how big is their class size, you will usually obtain an average rather higher than 30. Let’s take a stylised example to explain why. Say that the institution has class sizes of either ten or 50, and there are equal numbers of both class sizes. So the overall average class size is 30. But in selecting a random student, it is five times more likely that he or she will come from a class of 50 students than of ten students. So for every one student who replies ‘10’ to your enquiry about their class size, there will be five who answer ’50.’ So the average class size thrown up by your survey is 5×50 + 1 x 10, divided by 6. This equals 260/6 = 43.3. So the act of inspecting the class sizes actually increases the average obtained compared to the uninspected average. The only circumstance in which the inspected and uninspected average coincides is when every class size is equal.
The range of real-life cases where this occurs is almost boundless. For example, you visit the gym at a random time of day and ask a random sample of those who are there how long they normally exercise for. The answer you obtain will likely well exceed the average of all those who attend the gym that day because it is more likely that when you turn up you will come across those who exercise for a long time than a short time.
Once you know about the Inspection Paradox, the world and our perception of our place in it, is never quite the same again.
Exercise
You arrive at someone’s home and are ushered into the garden. You know that a train passes the end of the garden every half an hour on average but the trains are actually scheduled so that half pass by with an interval of a quarter of an hour and half with an interval of 45 minutes. Given that you have no clue when the last train passed by and the scheduled interval between that train and the next, how long can you expect to wait for the next train?
Links and References
Amir D. Aczel. Chance: A Guide to Gambling, Love, the Stock market and Just About Everything Else. 18 May, 2016. NY: Thunder’s Mouth Press.
On the Persistence of Bad Luck (and Good). Amir Aczel. Sept. 4, 2013. http://blogs.discovermagazine.com/crux/2013/09/04/on-the-persistence-of-bad-luck-and-good/#.XXJL0ihKh3g
The Waiting Time Paradox, or, Why is My Bus Always Late? https://jakevdp.github.io/blog/2018/09/13/waiting-time-paradox/
Probably Overthinking It. August 18, 2015. The Inspection Paradox is Everywhere. http://allendowney.blogspot.com/2015/08/the-inspection-paradox-is-everywhere.html
Further and deeper exploration of paradoxes and challenges of intuition and logic can be found in my recently published book, Probability, Choice and Reason.
One of the most celebrated pieces of correspondence in the history of probability and gambling, and one of which I am particularly fond, involves an exchange of letters between the greatest diarist of all time, Samuel Pepys, and the greatest scientist of all time, Sir Isaac Newton.
The six letters exchanged between Pepys in London and Newton in Cambridge related to a problem posed to Newton by Pepys about gambling odds. The interchange took place between November 22 and December 23, 1693. The ostensible reason for Mr. Pepys’ interest was to encourage the thirst for truth of his young friend, Mr. Smith. Whether Sir Isaac believed that tale or not we shall never know. The real reason, however, was later revealed in a letter written to a confidante by Pepys indicating that he himself was about to stake 10 pounds, a considerable sum in 1693, on such a bet. Now we’re talking!
The first letter to Newton introduced Mr. Smith as a fellow with a “general reputation…in this towne (inferiour to none, but superiour to most) for his maistery [of]…Arithmetick”.
What emerged has come down to us as the aptly named Newton-Pepys problem.
Essentially, the question came down to this:
Which of the following three propositions has the greatest chance of success.
- Six fair dice are tossed independently and at least one ‘6’ appears
- 12 fair dice are tossed independently and at least two ‘6’s appear.
- 18 fair dice are tossed independently and at least three ‘6’s appear.
Pepys was convinced that C. had the highest probability and asked Newton to confirm this.
Newton chose A as the highest probability, then B, then C, and produced his calculations for Pepys, who wouldn’t accept them.
So who was right? Newton or Pepys?
Well, let’s see.
The first problem is the easiest to solve.
What is the probability of A?
Probability that one toss of a coin produces a ‘6’ = 1/6
So probability that one toss of a coin does not produce a ‘6’ = 5/6
So probability that six independent tosses of a coin produces no ‘6’ = (5/6)6
So probability of AT LEAST one ‘6’ in 6 tosses = 1 – (5/6)6 = 0.6651
So far, so good.
The probability of problem B and probability of problem C are more difficult to calculate and involve use of the binomial distribution, though Newton derived the answers from first principles, by his method of ‘Progressions’.
Both methods give the same answer, but using the more modern binomial distribution is easier.
So let’s do it, along the way by introducing the idea of so-called ‘Bernoulli trials’.
The nice thing about a Bernoulli trial is that it has only two possible outcomes.
Each outcome can be framed as a ‘yes’ or ‘no’ question (success or failure).
Let probability of success = p.
Let probability of failure = 1-p.
Each trial is independent of the others and the probability of the two outcomes remains constant for every trial.
An example is tossing a coin. Will it lands heads?
Another example is rolling a die. Will it come up ‘6’?
Yes = success (S); No = failure (F).
Let probability of success, P (S) = p; probability of failure, P (F) = 1-p.
So the question: How many Bernoulli trials are needed to get to the first success?
This is straightforward, as the only way to need exactly five trials, for example, is to begin with four failures, i.e. FFFFS.
Probability of this = (1-p) (1-p) (1-p) (1-p) p = (1-p)4 p
Similarly, the only way to need exactly six trials is to begin with five failures, i.e. FFFFFS.
Probability of this = (1-p) (1-p) (1-p) (1-p) (1-p) p = (1-p)5 p
More generally, the probability that success starts on trial number n =
(1-p)n-1 p
This is a geometric distribution. This distribution deals with the number of trials required for a single success.
But what is the chance that the first success takes AT LEAST some number of trials, say 12 trials?
One method is to add the probability of 12 trials to prob. of 13 trials to prob. of 14 trials to prob. of 15 trials, etc. …………………………
Easier method: The only time you will need at least 12 trials is when the first 11 trials are all failures, i.e. (1-p)11
In a sequence of Bernoulli trials, the probability that the first success takes at least n trials is (1-p)n-1
Let’s take a couple of examples.
Probability that the first success (heads on coin toss) takes at least three trials (tosses of the coin)= (1-0.5)2 = 0.25
Probability that the first success (heads on coin toss) takes at least four trials (tosses of the coin)= (1-0.5)3 = 0.125
But so far we have only learned how to calculate the probability of one success in so many trials.
What if we want to know the probability of two, or three, or however many successes?
To take an example, what is the probability of exactly two ‘6’s in five throws of the die?
To determine this, we need to calculate the number of ways two ‘6’s can occur in five throws of the die, and multiply that by the probability of each of these ways occurring.
So, probability = number of ways something can occur multiplied by probability of each way occurring.
How many ways can we throw two ‘6’s in five throws of the die?
Where S = Success in throwing a ‘6’, F = Fail in throwing a ‘6’, we have:
SSFFF; SFSFF; SFFSF; SFFFS; FSSFF; FSFSF; FSFFS; FFSSF; FFSFS; FFFSS
So there are 10 ways of throwing two ‘6’s in five throws of the dice.
More formally, we are seeking to calculate how many ways 2 things can be chosen from 5. This is known as ‘5 Choose 2’, written as:
5 C 2= 10
More generally, the number of ways k things can be chosen from n is:
nC k = n! / (n-k)! k!
n! (known as n factorial) = n (n-1) (n-2) … 1
k! (known as k factorial) = k (k-1) (k-2) … 1
Thus, 5C 2 = 5! / 3! 2! = 5x4x3x2x1 / (3x2x1x2x1) = 5×4/(2×1) = 20/2=10
So what is the probability of throwing exactly two ‘6’s in five throws of the die, in each of these ten cases? p is the probability of success. 1-p is the probability of failure.
In each case, the probability = p.p.(1-p).(1-p).(1-p)
= p2 (1-p)3
Since there are 5 C 2 such sequences, the probability of exactly 2 ‘6’s =
10 p2 (1-p)3
Generally, in a fixed sequence of n Bernoulli trials, the probability of exactly r successes is:
nC r x pr (1-p) n-r
This is the binomial distribution. Note that it requires that the probability of success on each trial be constant. It also requires only two possible outcomes.
So, for example, what is the chance of exactly 3 heads when a fair coin is tossed 5 times?
5C 3 x (1/2)3 x (1/2)2 = 10/32 = 5/16
And what is the chance of exactly 2 sixes when a fair die is rolled five times?
5 C 2x (1/6)2 x (5/6)3 = 10 x 1/36 x 125/216 = 1250/7776 = 0.1608
So let’s now use the binomial distribution to solve the Newton-Pepys problem.
- What is the probability of obtaining at least one six with 6 dice?
- What is the probability of obtaining at least two sixes with 12 dice?
- What is the probability of obtaining at least three sizes with 18 dice?
First, what is the probability of no sixes with 6 dice?
P (no sixes with six dice) = n C x . (1/6)x . (5/6)n-x, x = 0,1,2,…,n
Where x is the number of successes.
So, probability of no successes (no sixes) with 6 dice =
n!/(n-k)!k! = 6!/(6-0)!0! x (1/6)0 . (5/6)6-0 = 6!/6! X 1 x 1 x (5/6)6 = (5/6)6
Note that: 0! = 1
Here’s the proof: n! = n. (n-1)!
At n=1, 1! = 1. (1-1)!
So 1 = 0!
So, where x is the number of sixes, probability of at least one six is equal to ‘1’ minus the probability of no sixes, which can be written as:
P (x≥ 1) = 1 – P(x=0) = 1 – (5/6)6 = 0.665 (to three decimal places).
i.e. probability of at least one six = 1 minus the probability of no sixes.
That is a formal solution to Part 1 of the Newton-Pepys Problem.
Now on to Part 2.
Probability of at least two sixes with 12 dice is equal to ‘1’ minus the probability of no sixes minus the probability of exactly one six.
This can be written as:
P (x≥2) = 1 – P(x=0) – P(x=1)
P(x=0) in 12 throws of the dice = (5/6)12
P (x=1) in 12 throws of the dice = 12 C 1 . (1/6)1 . (5/6)11nC k = n! / (n-k)! k!
So 12 C 1
= 12! / (12-1)! 1! = 12! / 11! 1! = 12
So, P (x≥2) = 1 – (5/6)12 – 12. (1/6) . (5/6)11
= 1 – 0.112156654 – 2 . (0.134587985) = 0.887843346 – 0.26917597 =
= 0.618667376 = 0.619 (to 3 decimal places)
This is a formal solution to Part 2 of the Newton-Pepys Problem.
Now on to Part 3.
Probability of at least three sixes with 18 dice is equal to ‘1’ minus the probability of no sixes minus the probability of exactly one six minus the probability of at exactly two sixes.
This can be written as:
P (x≥3) = 1 – P(x=0) – P(x=1) – P(x=2)
P(x=0) in 18 throws of the dice = (5/6)18
P (x=1) in 18 throws of the dice = 18 C 1 . (1/6)1 . (5/6)17
nC k = n! / (n-k)! k!
So 18 C 1
= 18! / (18-1)! 1! = 18
So P (x=1) = 18. (1/6)1 . (5/6)17
P (x=2) = 18 C 2 . (1/6)2 .(5/6)16
18 C 2
= 18! / (18-2)! 2! = 18!/16! 2! = 18. (17/2)
So P (x=2) = 18. (17/2) (1/6)2 (5/6)16
So P(x=3) = 1 – P (x=0) – (P(x=1) – P (x=2)
P (x=0) = (5/6)18
= 0.0375610365
P (x=1) = 18. 1/6. (0.0450732438) = 0.135219731
P (x=2) = 18. (17/2) (1/36) (0.0540878926) = 0.229873544
So P(x=3) = 1 – 0.0375610365 – 0.135219731 – 0.229873544 =
P(x≥3) = 0.597345689 = 0.597 (to 3 decimal places, )
This is a formal solution to Part 3 of the Newton-Pepys Problem.
So, to re-state the Newton-Pepys problem.
Which of the following three propositions has the greatest chance of success?
- Six fair dice are tossed independently and at least one ‘6’ appears.
- 12 fair dice are tossed independently and at least two ‘6’s appear.
- 18 fair dice are tossed independently and at least three ‘6’s appear.
Pepys was convinced that C. had the highest probability and asked Newton to confirm this.
Newton chose A, then B, then C, and produced his calculations for Pepys, who wouldn’t accept them.
So who was right? Newton or Pepys?
According to our calculations, what is the probability of A? 0.665
What is the probability of B? 0.619
What is the probability of C? 0.597
So Sir Isaac’s solution was right. Samuel Pepys was wrong, a wrong compounded by refusing to accept Newton’s solution. How much he lost gambling on his misjudgement is mired in the mists of history. The Newton-Pepys Problem is not, and continues to tease our brains to this very day.
References and Links
Newton and Pepys. DataGenetics. http://datagenetics.com/blog/february12014/index.html
Newton-Pepys problem. Wikipedia. https://en.wikipedia.org/wiki/Newton%E2%80%93Pepys_problem